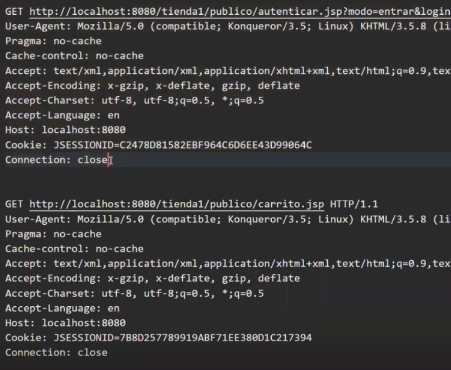
[python] 웹 공격 로그 분석 인공지능 학습 (기반 코드 + 프로젝트 설계)
oolongeya
·2021. 10. 31. 13:54
정상, 비정상 웹 로그 데이터 text 파일을 이용해서 (normal, anormal, test, train)
머신러닝 학습을 시킨 후 로그 분석으로 공격인지 아닌지를 분류하는 것이 목표.
데이터를 이용해서 (GET, POST 부분 확인 + url 기반)
아래와 같은 과정으로 진행한다.
파싱 -> 데이터 셋, 라벨링 (정상, 비정상 분류) -> 벡터 변환 (단어) -> 훈련 -> 테스트 |
1
2
3
4
5
6
7
8
9
10
11
12
13
14
15
16
17
18
19
20
21
22
23
24
25
26
27
28
29
30
31
32
33
34
35
36
37
38
39
40
41
42
43
44
45
46
47
48
49
50
51
52
53
54
55
56
57
58
59
60
61
62
63
64
65
66
67
68
69
|
import pandas as pd
import re
import numpy as np
from sklearn.feature_extraction.text import TfidfVectorizer
from sklearn.ensemble import RandomForestClassifier
from sklearn.metrics import accuracy_score,f1_score
def parsing(path):#파싱을 진행하는 함수
with open(path,'r',encoding='utf-8') as f:#파일을 읽어드리고 ['로그','로그',...] 이런식으로 로그를 구조화
train=[]
para=""
while True:
l = f.readline() #한줄씩 읽어 옵니다
if not l:
break #파일을 전부 읽으면 읽기를 중단합니다.
if l != "\n":
para +=l
else:
if para!='':
if para[:4]=='POST': #Method가 POST인 경우 예외적으로 바디까지 가져옵니다.
para+=f.readline()
train.append(para)
para=""
return train
def dataset(path,mod='train'): #데이터셋을 생성합니다. 파싱한 데이터와 라벨을 생성합니다
x = parsing(f'{path}norm_{mod}.txt') # mod에 따라 train을 가져올지 test 데이터를 가져올지 결정됩니다.
y = [0]*len(x) # 정상 라벨 0 을 정상 데이터 개수 만큼 생성
x += parsing(f'{path}anomal_{mod}.txt')
y += [1]*(len(x)-len(y)) # 비정상 라벨 1을 비정상 데이터 개수 만큼 생성
return x, y
def vectorize(train_x,test_x): #문장을 벡터로 만듭니다 해당 코드에서는 기본적인 tf idf를 사용하고 있습니다.
tf = TfidfVectorizer()
tf = tf.fit(train_x)
train_vec = tf.transform(train_x)
test_vec = tf.transform(test_x)
return train_vec,test_vec
def train(train_vec,train_y): #랜덤 포레스트로 훈련 시킵니다. 모델을 바꾸고 싶다면 이 함수를 변경해야 합니다.
rf = RandomForestClassifier()
rf.fit(train_vec,train_y)
return rf
def test(test_y,test_vec,rf): #입렵 받은 테스트와 모델로 테스트를 실시합니다
pred = rf.predict(test_vec)
print(accuracy_score(test_y,pred))
print(f1_score(test_y,pred))
return pred
############### 실행 코드 #######################
################################################
train_x, train_y = dataset('./','train')
test_x, test_y = dataset('./','test')
train_vec, test_vec = vectorize(train_x, test_x)
rf = train(train_vec, train_y)
pred = test(test_y,test_vec, rf)
########################################################
tf = TfidfVectorizer()
tf = tf.fit(train_x)
# print(len(tf.vocabulary_)) # 고유한 단어가 대략 8만개가 나옵니다
# print(tf.transform(train_x)[0]) #로그 하나당 약 8만차원이 나옵니다
# 필요없는 문장 때문에 단어의 개수가 많이 진것일 수도 있으니 데이터를 분석하여 필요한 부분만 사용하는것이 좋습니다
|
cs |
깃헙에서 프로젝트 진행
데이터 셋 파싱 코드 = py 파일로 작성
리포지토리에 csic 폴더를 만들고 데이터 셋을 저장
구현을 원하는 모델을 선택하고 해당 모드를 사용한 코드 = py 파일로 작성
summary ipynb 파일로 최종 저리
ex) 데이터 셋 파싱 코드 + SVM을 이용한 학습 예제 py 코드
1
2
3
4
5
6
7
8
9
10
11
12
13
14
15
16
17
18
19
20
21
22
23
24
25
26
27
28
29
30
31
32
33
34
35
36
37
38
39
40
41
42
43
44
45
46
47
48
49
50
51
52
53
54
55
56
57
58
59
60
61
62
63
64
65
66
|
import io
import urllib.parse
import numpy as np
def parse(file_in, file_out):
f = open("csic/" + file_in, 'r', encoding="utf8")
lines = list(map(lambda line: line.strip(), f.readlines()))
res = []
for i in range(len(lines)):
line = lines[i]
words = line.split(' ')
url_req = ''
is_req = False
if line.startswith("GET"):
is_req = True
url_req = words[0] + words[1]
elif line.startswith("POST") or line.startswith("PUT"):
is_req = True
url_req = words[0] + words[1]
idx = 1
while not lines[i + idx].startswith("Content-Length"):
idx += 1
url_req += '?' + lines[i + idx + 2]
if is_req:
res.append(url_req)
f.close()
out = io.open(file_out, 'w', encoding="utf-8")
for e in res:
out.writelines(urllib.parse.unquote(e).replace('\n', '').lower() + '\n')
print("Parsing complete.", len(res), "requests earned from", file_in)
def load_parsed(file):
with open(file, 'r', encoding="utf8") as f:
data = f.readlines()
ret = []
for i in data:
i = i.strip()
if i != '':
ret.append(i)
return ret
# 0: normal, 1: anomaly
def make_data_set(parsed: list, label: int):
return {
"data": parsed,
"target": np.array([label] * len(parsed), dtype=np.uint8),
"target_names": np.array(["normal", "anomaly"])
}
def combine_data_set(data_l: dict, data_r: dict):
if "target_names" not in data_l or "target_names" not in data_r:
print("Invalid data set!")
return False
if not np.array_equal(data_l["target_names"], data_r["target_names"]):
print("Invalid combining!")
return False
return {
"data": data_l["data"] + data_r["data"],
"target": np.append(data_l["target"], data_r["target"]),
"target_names": data_l["target_names"].copy()
}
|
cs |
1
2
3
4
5
6
7
8
9
10
11
12
13
14
15
16
17
18
19
20
21
22
23
24
25
26
27
28
29
30
31
32
33
34
35
36
37
38
39
40
41
42
43
44
45
46
47
48
49
50
51
52
|
import csic_parser
from sklearn.metrics import accuracy_score
from sklearn.feature_extraction.text import TfidfVectorizer
from sklearn.pipeline import Pipeline
from sklearn.preprocessing import StandardScaler
from sklearn.svm import SVC
anomaly_train_raw = "anomal_train.txt"
anomaly_test_raw = "anomal_test.txt"
normal_train_raw = "norm_train.txt"
normal_test_raw = "norm_test.txt"
csic_parser.parse(normal_train_raw, "normal_train.txt")
csic_parser.parse(normal_test_raw, "normal_test.txt")
csic_parser.parse(anomaly_train_raw, "anomaly_train.txt")
csic_parser.parse(anomaly_test_raw, "anomaly_test.txt")
normal_train = csic_parser.load_parsed("normal_train.txt")
normal_train = csic_parser.make_data_set(normal_train, 0)
anomaly_train = csic_parser.load_parsed("anomaly_train.txt")
anomaly_train = csic_parser.make_data_set(anomaly_train, 1)
train_data = csic_parser.combine_data_set(normal_train, anomaly_train)
normal_test = csic_parser.load_parsed("normal_test.txt")
normal_test = csic_parser.make_data_set(normal_test, 0)
anomaly_test = csic_parser.load_parsed("anomaly_test.txt")
anomaly_test = csic_parser.make_data_set(anomaly_test, 1)
test_data = csic_parser.combine_data_set(normal_test, anomaly_test)
vectorizer = TfidfVectorizer(
min_df=0,
analyzer="char",
sublinear_tf=True,
ngram_range=(3, 3)
)
X_train = train_data["data"]
y_train = train_data["target"]
X_test = test_data["data"]
y_test = test_data["target"]
vectorizer.fit(X_train)
X_train = vectorizer.transform(X_train)
X_test = vectorizer.transform(X_test)
svclassifier = SVC(kernel='linear', degree=8)
svclassifier.fit(X_train, y_train)
y_pred = svclassifier.predict(X_test)
score = accuracy_score(y_pred, y_test)
print("SVM 모델의 정확도: ", score)
|
cs |
반응형
'프로젝트 (Project) > 웹 로그 기반 웹 공격 탐지 분석 인공지능' 카테고리의 다른 글
[python] 웹 공격 로그 분석 인공지능 학습 (최종 산출물) (0) | 2021.11.19 |
---|---|
[python] 웹 공격 로그 분석 인공지능 학습 (프로젝트 코드) (0) | 2021.11.08 |